Recently, the team led by Associate Professor Cao Dongsheng from Xiangya School of Pharmaceutical Sciences, CSU, published an online treatise titled “Improving Structure-Based Virtual Screening Performance via Learning From Scoring Function Components” (DOI:10.1093/bib/bbaa094)inBriefings in Bioinformatics,an international journal providing an authoritative source of information in the field of bioinformatics, which is top-ranked by the JCR with an IF=9.101 insubject category 1.The study proposes a method to improve the virtual screening capability of molecular docking software with machine learning algorithm.
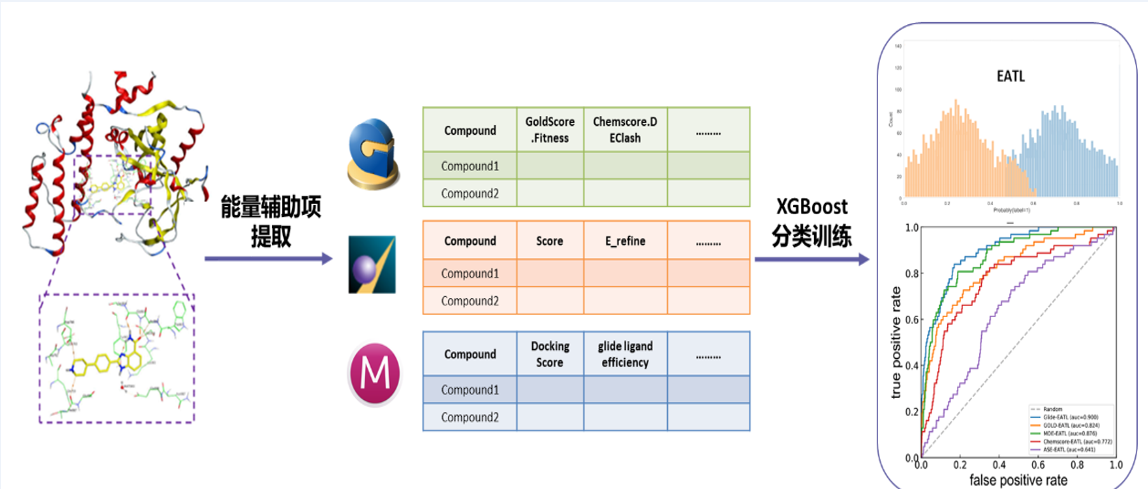
Molecular docking simulation is an important approach for structure-based drug design. Protein-ligand docking generally consists of two components, sampling and scoring. Sampling searches for the optimal binding between a small molecule (ligand) and a target macromolecule (protein) by continuously optimizing the position of the small molecule (placed in a protein binding pocket) and its flexible torsion angle. Scoring predicts the binding tightness with a physical or empirical energy function. Traditional scoring functions are usually trained to explore a linear relationship between predicted and experimental binding affinities, but this linear relationship does not always exist, so it cannot meet the actual needs. Improving the virtual screening capability of docking scoring functions can greatly save time and resource costs, and accelerate the discovery of lead compounds.
In this study, 61 scoring components were extracted of three docking programs (MOE, GOLD and Schrödinger) to represent protein ligand interactions and a classification model was trained using the XGBoost algorithm to predict the possible binding between a small molecule and a target macromolecule, so as to improve the virtual screening performance of the docking programs. Such model (a machine learning algorithm) was validated based on 8 diverse datasets and evaluated using multiple measures for virtual screening (ROC, BEDROC, logAUC, EF) to confirm its ability to significantly improve the efficiency of virtual screening based on docking and its superiority to other similar screening models. This model combines empirical knowledge with machine learning. It is easy to operate and can be extended to other docking programs that can provide energy functions.
The first author of this treatise is Xiong Guoli, a master candidate of year 2019 from Xiangya School of Pharmaceutical Sciences, and the corresponding authors are Associate Professor Cao Dongsheng and Professor Hou Tingjun from Zhejiang University. Besides, a series of papers on the molecular docking algorithm has been published recently in Briefings in Bioinformatics(IF=9.101, 2 articles), Journal of Chemical Information and Modeling(IF=3.966, 1 article), Journal of Chemical Theory and Computation(IF=5.313, 1 article), and Physical Chemistry Chemical Physics(IF=3.520, 1 article), of which the corresponding authors are Associate Professor Cao Dongsheng and Professor Hou Tingjun from Zhejiang University. All studies above were supported by the Fund for Major State Program for Basic Research by Young Scientists, and the National Natural Science Foundation of China (NSFC).
Source: Xiangya School of Pharmaceutical Sciences